Author: Daphne Koller
Edition: 1
Binding: Hardcover
ISBN: 0262013193
Edition: 1
Binding: Hardcover
ISBN: 0262013193
Probabilistic Graphical Models: Principles and Techniques (Adaptive Computation and Machine Learning series)
Most tasks require a person or an automated system to reason--to reach conclusions based on available information. Download Probabilistic Graphical Models: Principles and Techniques (Adaptive Computation and Machine Learning series) from rapidshare, mediafire, 4shared. The framework of probabilistic graphical models, presented in this book, provides a general approach for this task. The approach is model-based, allowing interpretable models to be constructed and then manipulated by reasoning algorithms. These models can also be learned automatically from data, allowing the approach to be used in cases where manually constructing a model is difficult or even impossible. Because uncertainty is an inescapable aspect of most real-world applications, the book focuses on probabilistic models, which make the uncertainty explicit and provide models that are more faithful to reality. Probabilistic Graphical Mo Search and find a lot of computer books in many category availabe for free download.
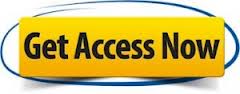
Probabilistic Graphical Models Free
Probabilistic Graphical Models computer books for free. The framework of probabilistic graphical models, presented in this book, provides a general approach for this task. The approach is model-based, allowing interpretable models to be constructed and then manipulated by reasoning algorithms. These models can also be learned automatically from data, allowing the approach to be used in cases where manually constructing a model is difficult or even impossible. Because uncertainty is an inescapable aspect of most real-world applications, the book focuses on probabilistic models, which make the uncertainty explicit and provide models that are more faithful to reality he framework of probabilistic graphical models, presented in this book, provides a general approach for this task. The approach is model-based, allowing interpretable models to be constructed and then manipulated by reasoning algorithms. These models can also be learned automatically from data, allowing the approach to be used in cases where manually constructing a model is difficult or even impossible. Because uncertainty is an inescapable aspect of most real-world applications, the book focuses on probabilistic models, which make the uncertainty explicit and provide models that are more faithful to reality. Probabilistic Graphical Mo
Related education books
Machine Learning: A Probabilistic Perspective (Adaptive Computation and Machine Learning series)
Today's Web-enabled deluge of electronic data calls for automated methods of data analysis. Machine learning provides these, developing methods that can automatically detect patterns in data and then use the uncovered patterns to predict future data.

Pattern Recognition and Machine Learning (Information Science and Statistics)
This is the first textbook on pattern recognition to present the Bayesian viewpoint. The book presents approximate inference algorithms that permit fast approximate answers in situations where exact answers are not feasible. It uses graphical models

Bayesian Reasoning and Machine Learning
Machine learning methods extract value from vast data sets quickly and with modest resources. They are established tools in a wide range of industrial applications, including search engines, DNA sequencing, stock market analysis, and robot locomotion

The Elements of Statistical Learning: Data Mining, Inference, and Prediction, Second Edition (Springer Series in Statistics)
During the past decade there has been an explosion in computation and information technology. With it have come vast amounts of data in a variety of fields such as medicine, biology, finance, and marketing. The challenge of understanding these data h

No comments:
Post a Comment